Cancer genetics and deep learning applications for diagnosis, prognosis, and categorization
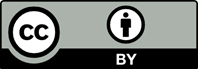
Gene expression data are used to discover meaningful hidden information in gene datasets. Cancer and other disorders may be diagnosed based on differences in gene expression profiles, and this information can be gleaned by gene sequencing. Thanks to the tremendous power of artificial intelligence (AI), healthcare has become a significant user of deep learning (DL) for predicting cancer diseases and categorizing gene expression. Gene expression Microarrays have been proved effective in predicting cancer diseases and categorizing gene expression. Gene expression datasets contain only limited samples, but the features of cancer are diverse and complex. To overcome their dimensionality, gene expression datasets must be enhanced. By learning and analyzing features of input data, it is possible to extract features, as multidimensional arrays, from the data. Synthetic samples are needed to strengthen the range of information. DL strategies may be used when gene expression data are used to diagnose and classify cancer diseases.
- Shen J, Shi J, Luo J, et al. Deep learning approach for cancer subtype classification using high-dimensional gene expression data. BMC Bioinformatics. 2022;23(1):430. doi: 10.1186/s12859-022-04980-9
- Khorshed T, Moustafa MN, Rafea A. Deep learning for multi-tissue cancer classification of gene expressions (GeneXNet). IEEE Access. 2020;8:90615-90629. doi: 10.1109/ACCESS.2020.2992907
- Zuo Z, Wang P, Chen X, Tian L, Ge H, Qian D. SWnet: A deep learning model for drug response prediction from cancer genomic signatures and compound chemical structures. BMC Bioinformatics. 2021;22(1):434. doi: 10.1186/s12859-021-04352-9
- Xiao Y, Wu J, Lin Z. Cancer diagnosis using generative adversarial networks based on deep learning from imbalanced data. Comput Biol Med. 2021;135:104540. doi: 10.1016/j.compbiomed.2021.104540
- Echle A, Rindtorff NT, Brinker TJ, Luedde T, Pearson AT, Kather JN. Deep learning in cancer pathology: A new generation of clinical biomarkers. Br J Cancer. 2021;124(4):686-696. doi: 10.1038/s41416-020-01122-x
- Gupta S, Gupta MK, Shabaz M, Sharma A. Deep learning techniques for cancer classification using microarray gene expression data. Front Physiol. 2022;13:952709. doi: 10.3389/fphys.2022.952709
- Majji R, Maram B, Rajeswari R. Chronological horse herd optimization-based gene selection with deep learning towards survival prediction using PAN-Cancer gene-expression data. Biomed Signal Process Control. 2023;84:104696. doi: 10.1016/j.bspc.2023.104696
- Almarzouki HZ. Deep-learning-based cancer profiles classification using gene expression data profile. J Healthc Eng. 2022;2022:4715998. doi: 10.1155/2022/4715998
- Khorshed T, Moustafa MN, Rafea A, editors. Multi-tissue Cancer Classification of Gene Expressions using Deep Learning. In: 2020 IEEE Sixth International Conference on Big Data Computing Service and Applications (BigDataService). IEEE; 2020. doi: 10.1109/BigDataService49289.2020.00027
- Khorshed T, Moustafa MN, Rafea A, editors. Learning and Visualizing Genomic Signatures of Cancer Tumors Using Deep Neural Networks. In: 2020 International Joint Conference on Neural Networks (IJCNN). IEEE; 2020. doi: 10.1109/IJCNN48605.2020.9207368
- Mallik S, Seth S, Bhadra T, Zhao Z. A Linear regression and deep learning approach for detecting reliable genetic alterations in cancer using DNA Methylation and Gene expression data. Genes (Basel). 2020;11(8):931. doi: 10.3390/genes11080931
- Rezaee K, Jeon G, Khosravi MR, Attar HH, Sabzevari A. Deep learning-based microarray cancer classification and ensemble gene selection approach. IET Syst Biol. 2022;16(3-4):120-131. doi: 10.1049/syb2.12044
- Wang S, Zhang H, Liu Z, Liu Y. A novel deep learning method to predict lung cancer long-term survival with biological knowledge incorporated gene expression images and clinical data. Front Genet. 2022;13:800853. doi: 10.3389/fgene.2022.800853
- Hanczar B, Bourgeais V, Zehraoui F. Assessment of deep learning and transfer learning for cancer prediction based on gene expression data. BMC Bioinformatics. 2022;23(1):262. doi: 10.1186/s12859-022-04807-7
- Liu S, Yao W. Prediction of lung cancer using gene expression and deep learning with KL divergence gene selection. BMC Bioinformatics. 2022;23(1):175. doi: 10.1186/s12859-022-04689-9
- Zhao Y, Zhou Y, Liu Y, et al. Uncovering the prognostic gene signatures for the improvement of risk stratification in cancers by using deep learning algorithm coupled with wavelet transform. BMC Bioinformatics. 2020;21(1):195. doi: 10.1186/s12859-020-03544-z
- Orrico KB. Basic concepts of cancer genetics and receptor tyrosine kinase inhibition for pharmacists. A narrative review. J Oncol Pharm Pract. 2023;29(5):1187-1195. doi: 10.1177/10781552231153814
- Pettini F, Visibelli A, Cicaloni V, Iovinelli D, Spiga O. Multi-omics model applied to cancer genetics. Int J Mol Sci. 2021;22(11):5751. doi: 10.3390/ijms22115751
- Ferguson LR, Chen H, Collins AR, et al. Genomic instability in human cancer: Molecular insights and opportunities for therapeutic attack and prevention through diet and nutrition. Semin Cancer Biol. 2015;35(Suppl):S5-S24. doi: 10.1016/j.semcancer.2015.03.005
- Hesson LB, Hitchins MP, Ward RL. Epimutations and cancer predisposition: Importance and mechanisms. Curr Opin Genet Dev. 2010;20(3):290-298. doi: 10.1016/j.gde.2010.02.005
- Hassanpour SH, Dehghani M. Review of cancer from perspective of molecular. J Cancer Res Pract. 2017;4(4):127-129. doi: 10.1016/j.jcrpr.2017.07.001
- Seligson DB, Horvath S, Shi T, et al. Global histone modification patterns predict risk of prostate cancer recurrence. Nature. 2005;435(7046):1262-1266. doi: 10.1038/nature03672
- Quail DF, Joyce JA. Microenvironmental regulation of tumor progression and metastasis. Nat Med. 2013;19(11):1423-1437. doi: 10.1038/nm.3394
- Turnbull C, Sud A, Houlston RS. Cancer genetics, precision prevention and a call to action. Nat Genet. 2018;50(9):1212-1218. doi: 10.1038/s41588-018-0202-0
- De Anda-Jáuregui G, Hernández-Lemus E. Computational oncology in the multi-omics era: State of the Art. Front Oncol. 2020;10:423. doi: 10.3389/fonc.2020.00423
- Ergin S, Kherad N, Alagoz M. RNA sequencing and its applications in cancer and rare diseases. Mol Biol Rep. 2022;49(3):2325-2333. doi: 10.1007/s11033-021-06963-0
- Mortezaei Z. Computational methods for analyzing RNA sequencing contaminated samples and its impact on cancer genome studies. Inform Med Unlocked. 2022;32:101054. doi: 10.1016/j.imu.2022.101054
- Sezer A. A review on microarray technology in molecular diagnostics. Bioeng Stud. 2021;2(2):7-11. doi: 10.37868/bes.v2i2.id191
- Cowell JK, Hawthorn L. The application of microarray technology to the analysis of the cancer genome. Curr Mol Med. 2007;7(1):103-120. doi: 10.2174/156652407779940387
- Koch M, Wiese M. Accessing cancer metabolic pathways by the use of microarray technology. Curr Pharm Des. 2013;19(4):790-805.
- Li L, Li L, Sun Q. High expression of cuproptosis-related SLC31A1 gene in relation to unfavorable outcome and deregulated immune cell infiltration in breast cancer: An analysis based on public databases. BMC Bioinformatics. 2022;23(1):350. doi: 10.1186/s12859-022-04894-6
- Zhong Z, Hong M, Chen X, et al. Transcriptome analysis reveals the link between lncRNA-mRNA co-expression network and tumor immune microenvironment and overall survival in head and neck squamous cell carcinoma. BMC Med Genomics. 2020;13(1):57. doi: 10.1186/s12920-020-0707-0
- Athar A, Füllgrabe A, George N, et al. ArrayExpress update - From bulk to single-cell expression data. Nucleic Acids Res. 2018;47(D1):D711-D715. doi: 10.1093/nar/gky964
- Rhodes DR, Yu J, Shanker K, et al. ONCOMINE: A cancer microarray database and integrated data-mining platform. Neoplasia. 2004;6(1):1-6. doi: 10.1016/S1476-5586(04)80047-2
- Nwosu IO, Piccolo SR. A systematic review of datasets that can help elucidate relationships among gene expression, race, and immunohistochemistry-defined subtypes in breast cancer. Cancer Biol Ther. 2021;22(7-9):417-429. doi: 10.1080/15384047.2021.1953902
- Alameer A, Chicco D. geoCancerPrognosticDatasetsRetriever: A bioinformatics tool to easily identify cancer prognostic datasets on Gene Expression Omnibus (GEO). Bioinformatics. 2022;38(6):1761-1763. doi: 10.1093/bioinformatics/btab852
- Zenbout I, Meshoul S, editors. Advanced Machine Learning Models for Large Scale Gene Expression Analysis in Cancer Classification: Deep Learning Versus Classical Models. In: Big Data, Cloud and Applications: Third International Conference, BDCA. Revised Selected Papers 3. Kenitra, Morocco: Springer; 2018.
- Pirooznia M, Yang JY, Yang MQ, Deng Y. A comparative study of different machine learning methods on microarray gene expression data. BMC Genomics. 2008;9(1):S13. doi: 10.1186/1471-2164-9-S1-S13
- Alshareef AM, Alsini R, Alsieni M, et al. Optimal deep learning enabled prostate cancer detection using microarray gene expression. J Healthc Eng. 2022;2022:7364704. doi: 10.1155/2022/7364704
- Bhonde SB, Prasad JR, editors. Deep Learning Techniques in Cancer Prediction Using Genomic Profiles. In: 2021 6th International Conference for Convergence in Technology (I2CT). Maharashtra, India; 2021. doi: 10.1109/I2CT51068.2021.9417985
- Su AI, Welsh JB, Sapinoso LM, et al. Molecular classification of human carcinomas by use of gene expression signatures. Cancer Res. 2001;61(20):7388-7393.
- Bolón-Canedo V, Sánchez-Maroño N, Alonso-Betanzos A, Benítez JM, Herrera F. A review of microarray datasets and applied feature selection methods. Inf Sci. 2014;282:111-135. doi: 10.1016/j.ins.2014.05.042
- Wang S, Wei J. Feature selection based on measurement of ability to classify subproblems. Neurocomputing. 2017;224:155-165. doi: 10.1016/j.neucom.2016.10.062
- Han D, Kim J. Unified simultaneous clustering and feature selection for unlabeled and labeled data. IEEE Trans Neural Netw Learn Syst. 2018;29(12):6083-6098. doi: 10.1109/TNNLS.2018.2818444
- Perera K, Chan J, Karunasekera S, editors. Feature Selection for Multiclass Binary data. In: Advances in Knowledge Discovery and Data Mining: 22nd Pacific-Asia Conference, PAKDD 2018, Melbourne, VIC, Australia, Proceedings, Part III 22. Springer; 2018. doi: 10.1007/978-3-319-93040-4_5
- Araújo D, Neto AD, Martins A, Melo J, editors. Comparative Study on Dimension Reduction Techniques for Cluster analysis of Microarray Data. In: The 2011 International Joint Conference on Neural Networks. IEEE; 2011. doi: 10.1109/IJCNN.2011.6033447
- Sardana M, Agrawal RK, editors. A Comparative Study of Clustering Methods for Relevant Gene Selection in Microarray Data. In: Advances in Computer Science, Engineering and Applications: Proceedings of the Second International Conference on Computer Science, Engineering and Applications (ICCSEA 2012). Vol. 1. New Delhi, India: Springer; 2012.
- Sirinukunwattana K, Savage RS, Bari MF, Snead DR, Rajpoot NM. Bayesian hierarchical clustering for studying cancer gene expression data with unknown statistics. PLoS One. 2013;8(10):e75748. doi: 10.1371/journal.pone.0075748
- Li J, Liu R, Zhang M, Li Y, editors. Ensemble-based Multi-objective Clustering Algorithms for Gene Expression Data Sets. In: 2017 IEEE Congress on Evolutionary Computation (CEC). IEEE; 2017. doi: 10.1109/CEC.2017.7969331
- Liu S, Zhang J, Xiang Y, Zhou W, Xiang D. A study of data pre-processing techniques for imbalanced biomedical data classification. Int J Bioinform Res Appl. 2020;16(3):290-318.
- Sharma A, Rani R. An optimized framework for cancer classification using deep learning and genetic algorithm. J Med Imaging Health Inform. 2017;7(8):1851-1856. doi: 10.1166/jmihi.2017.2266
- Sun KF, Sun LM, Zhou D, et al. XGBG: A novel method for identifying ovarian carcinoma susceptible genes based on deep learning. Front Oncol. 2022;12:897503. doi: 10.3389/fonc.2022.897503
- Alharbi F, Vakanski A. Machine learning methods for cancer classification using gene expression data: A review. Bioengineering (Basel). 2023;10(2):173. doi: 10.3390/bioengineering10020173
- Liu R, Liu Y, Yan Y, Wang JY. Iterative deep neighborhood: A deep learning model which involves both input data points and their Neighbors. Comput Intell Neurosci. 2020;2020:9868017. doi: 10.1155/2020/9868017
- Young JD, Cai C, Lu X. Unsupervised deep learning reveals prognostically relevant subtypes of glioblastoma. BMC Bioinformatics. 2017;18(Suppl 11):381. doi: 10.1186/s12859-017-1798-2
- Abdullah AA, Hassan MM, Mustafa YT. A review on bayesian deep learning in healthcare: Applications and challenges. IEEE Access. 2022;10:36538-36562. doi: 10.1109/access.2022.3163384
- Alakwaa FM, Chaudhary K, Garmire LX. Deep learning accurately predicts estrogen receptor status in breast cancer metabolomics data. J Proteome Res. 2018;17(1):337-347. doi: 10.1021/acs.jproteome.7b00595
- Sokhangouy SK, Zeinali M, Nazari E, et al. Deep Learning Assisted Identification of ATP5J and ALDH1A2 Combination in RNA-Sequencing Data as a Novel Specific Potential Diagnostic Biomarker in Prostate Cancer. PREPRINT (Version 1); 2023. Available from: https://researchsquare. doi: 10.21203/rs.3.rs-3482392/v1
- Sur C. GSIAR: Gene-subcategory interaction-based improved deep representation learning for breast cancer subcategorical analysis using gene expression, applicable for precision medicine. Med Biol Eng Comput. 2019;57:2483-2515. doi: 10.1007/s11517-019-02038-2
- LeCun Y, Bengio Y, Hinton G. Deep learning. Nature. 2015;521(7553):436-444. doi: 10.1038/nature14539
- Inoue M, Inoue S, Nishida T. Deep recurrent neural network for mobile human activity recognition with high throughput. Artif Life Robot. 2018;23(2):173-185. doi: 10.1007/s10015-017-0422-x
- Eluri NR, Kancharla GR, Dara S, Dondeti V. Cancer data classification by quantum-inspired immune clone optimization-based optimal feature selection using gene expression data: Deep learning approach. Data Technol Appl. 2022;56(2):247- 282. doi: 10.1108/DTA-05-2020-0109
- Yosinski J, Clune J, Bengio Y, Lipson H. How transferable are features in deep neural networks? Adv Neural Inf Process Syst. 2014;27:3320-3328. doi: 10.48550/arXiv.1411.1792
- Min S, Lee B, Yoon S. Deep learning in bioinformatics. Brief Bioinform. 2017;18(5):851-869. doi: 10.1093/bib/bbw068
- Bhat RR, Viswanath V, Li X, editors. DeepCancer: Detecting Cancer Via Deep Generative Learning Through Gene Expressions. In: 2017 IEEE 15th International Conference on Dependable, Autonomic and Secure Computing, 15th International Conference on Pervasive Intelligence and Computing, 3rd International Conference on Big Data Intelligence and Computing and Cyber Science and Technology Congress (DASC/PiCom/DataCom/CyberSciTech). IEEE; 2017. doi: 10.1109/DASC-PICom-DataCom-CyberSciTec.2017.152
- Tapak L, Ghasemi MK, Afshar S, Mahjub H, Soltanian A, Khotanlou H. Identification of gene profiles related to the development of oral cancer using a deep learning technique. BMC Med Genomics. 2023;16(1):35. doi: 10.1186/s12920-023-01462-6
- Majumder S, Pal V, Yadav A, Chakrabarty A. Performance analysis of deep learning models for binary classification of cancer gene expression data. J Healthc Eng. 2022;2022:1122536. doi: 10.1155/2022/1122536
- Tabares-Soto R, Orozco-Arias S, Romero-Cano V, Bucheli VS, Rodríguez-Sotelo JL, Jiménez-Varón CF. A comparative study of machine learning and deep learning algorithms to classify cancer types based on microarray gene expression data. PeerJ Comput Sci. 2020;6:e270. doi: 10.7717/peerj-cs.270
- Singh D, Febbo PG, Ross K, et al. Gene expression correlates of clinical prostate cancer behavior. Cancer Cell. 2002;1(2):203-209. doi: 10.1016/s1535-6108(02)00030-2
- Shah SH, Iqbal MJ, Ahmad I, Khan S, Rodrigues SJPC. Optimized Gene Selection and Classification of Cancer from Microarray Gene Expression Data Using Deep Learning. Neural Computing and Applications. Berlin: Springer; 2020. doi: 10.1007/s00521-020-05367-8
- Park S, Huang E, Ahn T. Classification and functional analysis between cancer and normal tissues using explainable pathway deep learning through RNA-sequencing gene expression. Int J Mol Sci. 2021;22(21):11531. doi: 10.3390/ijms222111531
- De Guia JM, Devaraj M, Leung CK, editors. DeepGx: Deep Learning Using Gene Expression for Cancer Classification. In: 2019 IEEE/ACM International Conference on Advances in Social Networks Analysis and Mining (ASONAM). IEEE; 2019. doi: 10.1145/3341161.3343516
- Ibrahim M, Muhammad Q, Zamarud A, Eiman H, Fazal F. Navigating glioblastoma diagnosis and care: Transformative pathway of artificial intelligence in integrative oncology. Cureus. 2023;15(8):e44214. doi: 10.7759/cureus.44214
- Chow JCL, Wong V, Li K. Generative Pre-trained transformer-empowered healthcare conversations: Current trends, challenges, and future directions in large language model-enabled medical chatbots. BioMedInformatics. 2024;4(1):837-852. doi: 10.3390/biomedinformatics4010047
- Ness S, Xuan TR, Oguntibeju OO. Influence of AI: Robotics in healthcare. Asian J Res Comput Sci. 2024;17(5):222-237. doi: 10.9734/ajrcos/2024/v17i5451
- Odah M. Artificial Intelligence (AI) and Machine Learning (ML) in Diagnosing Cancer: Current Trends. Preprints; 2024. p. 2024030433. doi: 10.20944/preprints202403.0433.v1
- Anyanwu EC, Okongwu CC, Olorunsogo TO, Ayo-Farai O, Osasona F, Daraojimba OD. Artificial intelligence in healthcare: A review of ethical dilemmas and practical applications. Int Med Sci Res J. 2024;4(2):126-140. doi: 10.51594/imsrj.v4i2.755
- Sharma R. Artificial intelligence in healthcare: A review. Turk J Comput Math Educ (TURCOMAT). 2020;11(1):1663-1667. doi: 10.61841/turcomat.v11i1.14628
- Esmaeilzadeh P. Use of AI-based tools for healthcare purposes: A survey study from consumers’ perspectives. BMC Med Inform Decis Mak. 2020;20(1):170. doi: 10.1186/s12911-020-01191-1
- Vallverdú J. Challenges and controversies of generative AI in medical diagnosis. Euphyía Rev Filos. 2023;17(32):88-121. doi: 10.33064/32euph4957
- Klemt C, Uzosike AC, Cohen-Levy WB, Harvey MJ, Subih MA, Kwon YM. The ability of deep learning models to identify total hip and knee arthroplasty implant design from plain radiographs. J Am Acad Orthop Surg. 2022;30(9):409-415. doi: 10.5435/JAAOS-D-21-00771
- Zhang J, Li Z, Lin H, et al. Deep learning assisted diagnosis system: Improving the diagnostic accuracy of distal radius fractures. Front Med (Lausanne). 2023;10:1224489. doi: 10.3389/fmed.2023.1224489
- Ando H, Chang H. A model of computing with road traffic dynamics. Nonlinear Theory Appl IEICE. 2021;12(2):175-180. doi: 10.1587/nolta.12.175
- Justus D, Brennan J, Bonner S, McGough AS. Predicting the Computational Cost of Deep Learning Models. In: 2018 IEEE International Conference on Big Data (Big Data). Seattle, WA, USA; 2018. doi: 10.1109/BigData.2018.8622396
- Purushotham S, Meng C, Che Z, Liu Y. Benchmarking deep learning models on large healthcare datasets. J Biomed Inform. 2018;83:112-134. doi: 10.1016/j.jbi.2018.04.007
- Bolhasani H, Jassbi SJ. Deep learning accelerators: A case study with MAESTRO. J Big Data. 2020;7(1):100. doi: 10.1186/s40537-020-00377-8
- Che Z, Purushotham S, Khemani R, Liu Y. Distilling knowledge from Deep Networks with applications to Healthcare Domain. arXiv; 2015. Available from: https://arxiv.org/abs/1512.03542
- Ali A, Ali H, Saeed A, et al. Blockchain-powered healthcare systems: Enhancing scalability and security with hybrid deep learning. Sensors (Basel). 2023;23(18):7740. doi: 10.3390/s23187740
- Bebortta S, Tripathy SS, Basheer S, Chowdhary CL. DeepMist: Toward deep learning assisted mist computing framework for managing healthcare big data. IEEE Access. 2023;11:42485-42496. doi: 10.1109/access.2023.3266374
- Baharani M, Biglarbegian M, Parkhideh B, Tabkhi H. Realtime deep learning at the edge for scalable reliability modelling of Si-MOSFET power electronics converters. IEEE Internet Things J. 2019;6(5):7375-7385. doi: 10.1109/jiot.2019.2896174
- Nguyen AT, Drealan MW, Luu DK, et al. A portable, selfcontained neuroprosthetic hand with deep learning-based finger control. J Neural Eng. 2021;18(5):056051. doi: 10.1088/1741-2552/ac2a8d
- Hoang D, Hoang S. Deep learning - cancer genetics and application of deep learning to cancer oncology. Vietnam J Sci Technol. 2022;60(6):885-928. doi: 10.15625/2525-2518/17256
- Lai YH, Chen WN, Hsu TC, Lin C, Tsao Y, Wu S. Overall survival prediction of non-small cell lung cancer by integrating microarray and clinical data with deep learning. Sci Rep. 2020;10(1):4679. doi: 10.1038/s41598-020-61588-w
- Zhu W, Xie L, Han J, Guo X. The application of deep learning in cancer prognosis prediction. Cancers (Basel). 2020;12(3):603. doi: 10.3390/cancers12030603
- Meng C, Trinh L, Xu N, Enouen J, Liu Y. Interpretability and fairness evaluation of deep learning models on MIMIC-IV dataset. Sci Rep. 2022;12(1):7166. doi: 10.1038/s41598-022-11012-2
- Miotto R, Wang F, Wang S, Jiang X, Dudley JT. Deep learning for healthcare: Review, opportunities and challenges. Brief Bioinform. 2018;19(6):1236-1246. doi: 10.1093/bib/bbx044
- Hage Chehade A, Abdallah N, Marion JM, Oueidat M, Chauvet P. Lung and colon cancer classification using medical imaging: A feature engineering approach. Phys Eng Sci Med. 2022;45(3):729-746. doi: 10.1007/s13246-022-01139-x
- Kiran Sree P. Deep learning for heart attack prediction. Biomed J Sci Tech Res. 2023;54(2):45718-45721. doi: 10.26717/bjstr.2023.54.008522
- Saillard C, Schmauch B, Laifa O, et al. Predicting survival after hepatocellular carcinoma resection using deep learning on histological slides. Hepatology. 2020;72(6):2000-2013. doi: 10.1002/hep.31207
- Wang S, Yang DM, Rong R, et al. Artificial intelligence in lung cancer pathology image analysis. Cancers ( Basel). 2019;11(11):1673. doi: 10.3390/cancers11111673
- Verma DDK. Explainable AI in healthcare: Interpretable deep learning models for disease diagnosis. Pharma Innov. 2019;8(3):561-565. doi: 10.22271/tpi.2019.v8.i3j.25392
- Wei P. Radiomics, deep learning and early diagnosis in oncology. Emerg Top Life Sci. 2021;5(6):829-835. doi: 10.1042/ETLS20210218
- Wulczyn E, Steiner DF, Xu Z, et al. Deep learning-based survival prediction for multiple cancer types using histopathology images. PLoS One. 2020;15(6):e0233678. doi: 10.1371/journal.pone.0233678
- Tran KA, Kondrashova O, Bradley A, Williams ED, Pearson JV, Waddell N. Deep learning in cancer diagnosis, prognosis and treatment selection. Genome Med. 2021;13(1):152. doi: 10.1186/s13073-021-00968-x
- Hosny A, Parmar C, Coroller TP, et al. Deep learning for lung cancer prognostication: A retrospective multi-cohort radiomics study. PLoS Med. 2018;15(11):e1002711. doi: 10.1371/journal.pmed.1002711
- Akazawa M, Hashimoto K. Development and Validation of Machine Learning Models for the Prediction of Overall Survival and Cancer-Specific Survival in Patients with Endometrial Cancer: An Analysis of the Surveillance, Epidemiology, and End Results (SEER) Database. Available from: https://ssrn. com/abstract=4191367