Electroencephalogram-based time-frequency analysis for Alzheimer’s disease detection using machine learning
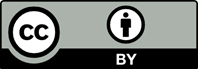
Background: Alzheimer’s disease (AD) is the most common form of dementia. The lack of effective prevention or cure makes AD a significant concern, as it is a progressive disease with symptoms that worsen over time. Objective: The aim of this study is to develop an algorithm capable of differentiating between patients with early-stage AD (mild cognitive impairment [MCI]), moderate AD, and healthy controls (C) using electroencephalogram (EEG) signals. Methods: A publicly available EEG database was utilized, with seven EEG recordings selected from each study group (MCI, AD, and C) to ensure a balanced dataset. For each 1-s segment of EEG data, 43 time-frequency features were computed. These features were then compressed over time using 10 statistical measures. Subsequently, 15 classifiers were employed to distinguish between paired groups using a 7-fold cross-validation. Results: The strategy yielded better results than state-of-the-art methods, achieving a 100% accuracy in both C versus MCI and C versus AD binary classifications. This improvement translated to a 2% increase in accuracy for C versus MCI and a 4% increase for C versus AD, despite a 1.2% decrease in performance for AD versus MCI. In addition, the proposed method outperformed prior work on the same database by 4.8% for the AD versus MCI comparison. Conclusion: The present study highlights the potential of EEG as a promising tool for early AD diagnosis. Nevertheless, a more extensive database should be used to enhance the generalizability of the results in future work.
- Azad NA, Al Bugami M, Loy-English I. Gender differences in dementia risk factors. Gend Med. 2007;4(2):120-129. doi: 10.1016/s1550-8579(07)80026-x
- Blennow K, de Leon MJ, Zetterberg H. Alzheimer’s disease. Lancet. 2006;368(9533):387-403. doi: 10.1016/S0140-6736(06)69113-7
- Petersen RC, Caracciolo B, Brayne C, Gauthier S, Jelic V, Fratiglioni L. Mild cognitive impairment: A concept in evolution. J Intern Med. 2014;275(3):214-228. doi: 10.1111/joim.12190
- Knopman DS, Petersen RC. Mild cognitive impairment and mild dementia: A clinical perspective. Mayo Clin Proc. 2014;89(10):1452-1459. doi: 10.1016/j.mayocp.2014.06.019
- Peters KR, Katz S. Voices from the field: Expert reflections on mild cognitive impairment. Dementia (London). 2015;14(3):285-297. doi: 10.1177/1471301214562135
- Reisberg B, Ferris SH, de Leon MJ, et al. Stage-specific behavioral, cognitive, and in vivo changes in community residing subjects with age-associated memory impairment and primary degenerative dementia of the Alzheimer type. Drug Dev Res. 1988;15(2-3):101-114. doi: 10.1002/ddr.430150203
- Petersen RC, Smith GE, Waring SC, Ivnik RJ, Kokmen E, Tangelos EG. Aging, memory, and mild cognitive impairment. Int Psychogeriatr. 1997;9 Suppl 1:65-69. doi: 10.1017/s1041610297004717
- Jack CR Jr., Andrews SJ, Beach TG, et al. Revised criteria for the diagnosis and staging of Alzheimer’s disease. Nat Med. 2024;30(8):2121-2124. doi: 10.1038/s41591-024-02988-7
- Tahami Monfared AA, Byrnes MJ, White LA, Zhang Q. Alzheimer’s disease: Epidemiology and clinical progression. Neurol Ther. 2022;11(2):553-569. doi: 10.1007/s40120-022-00338-8
- Porsteinsson AP, Isaacson RS, Knox S, Sabbagh MN, Rubino I. Diagnosis of early Alzheimer’s disease: Clinical practice in 2021. J Prev Alzheimers Dis. 2021;8(3):371-386. doi: 10.14283/jpad.2021.23
- McKhann G, Drachman D, Folstein M, Katzman R, Price D, Stadlan EM. Clinical diagnosis of Alzheimer’s disease: Report of the NINCDS-ADRDA Work Group under the auspices of Department of Health and Human Services Task Force on Alzheimer’s Disease. Neurology. 1984;34(7):939-944. doi: 10.1212/wnl.34.7.939
- Dubois B, Feldman HH, Jacova C, et al. Research criteria for the diagnosis of Alzheimer’s disease: Revising the NINCDS-ADRDA criteria. Lancet Neurol. 2007;6(8):734-746. doi: 10.1016/S1474-4422(07)70178-3
- Loewenstein D, Acevedo A, Ownby R, Schram L. The NINCDS-ADRDA neuropsychological criteria for Alzheimer’s disease: A confirmatory factor analysis of single versus multi-factor models. Arch Clin Neuropsychol. 2000;15(8):747-747. doi: 10.1093/arclin/15.8.747
- Gillis C, Mirzaei F, Potashman M, Ikram MA, Maserejian N. The incidence of mild cognitive impairment: A systematic review and data synthesis. Alzheimers Dement (Amst). 2019;11(1):248-256. doi: 10.1016/j.dadm.2019.01.004
- Miltiadous A, Tzimourta KD, Afrantou T, et al. A dataset of scalp EEG recordings of Alzheimer’s disease, frontotemporal dementia and healthy subjects from routine EEG. Data. 2023;8(6):95. doi: 10.3390/data8060095
- Zheng X, Wang B, Liu H, et al. Diagnosis of Alzheimer’s disease via resting-state EEG: Integration of spectrum, complexity, and synchronization signal features. Front Aging Neurosci. 2023;15:1288295. doi: 10.3389/fnagi.2023.1288295
- Gevins AS, Rémond A. Methods of Analysis of Brain Electrical and Magnetic Signals. Netherlands: Elsevier; 1987.
- Lin Y, Tsao Y, Hsieh PJ. Neural correlates of individual differences in predicting ambiguous sounds comprehension level. Neuroimage. 2022;251:119012. doi: 10.1016/j.neuroimage.2022.119012
- Sanei S, Chambers JA. EEG Signal Processing. United States: Wiley; 2013.
- Silva G, Alves M, Cunha R, Bispo BC, Oliveira-Silva P, Rodrigues PM. Early detection of Alzheimer’s and Parkinson’s diseases using multiband nonlinear EEG analysis. Psychol Neurosci. 2022;15(4):360-374. doi: 10.1037/pne0000287
- Jeong J. EEG dynamics in patients with Alzheimer’s disease. Clin Neurophysiol. 2004;115(7):1490-1505. doi: 10.1016/j.clinph.2004.01.001
- Cejnek M, Vysata O, Valis M, Bukovsky I. Novelty detection-based approach for Alzheimer’s disease and mild cognitive impairment diagnosis from EEG. Med Biol Eng Comput. 2021;59(11-12):2287-2296. doi: 10.1007/s11517-021-02427-6
- Shahbakhti M, Beiramvand M, Hakimi N, et al. Fusing fuzzy entropy with gaussian and exponential membership functions outperforms traditional entropy metrics in monitoring the depth of anesthesia using a single frontal EEG channel. IEEE Sensors Lett. 2024;8(3):1-4. doi: 10.1109/lsens.2024.3369318
- Ahmadieh H, Ghassemi F. Assessing the effects of Alzheimer disease on EEG signals using the entropy measure: A meta-analysis. Basic Clin Neurosci. 2022;13(2):153-164. doi: 10.32598/bcn.2021.1144.3
- Mootoo XS, Fours A, Dinesh C, Ashkani M, Kiss A, Faltyn M. Detecting Alzheimer disease in EEG data with machine learning and the graph discrete fourier transform. medRxiv. 2023. doi: 10.1101/2023.11.01.23297940
- Vempati R, Sharma LD. A systematic review on automated human emotion recognition using electroencephalogram signals and artificial intelligence. Results Eng. 2023;18:101027. doi: 10.1016/j.rineng.2023.101027
- Rodrigues PM, Freitas DR, Teixeira JP, Alves D, Garrett C. Electroencephalogram signal analysis in Alzheimer’s disease early detection. Int J Reliable Qual E-Healthc. 2018;7(1):40-59. doi: 10.4018/ijrqeh.2018010104
- Wang R, Wang J, Yu H, Wei X, Yang C, Deng B. Power spectral density and coherence analysis of Alzheimer’s EEG. Cogn Neurodyn. 2015;9(3):291-304. doi: 10.1007/s11571-014-9325-x
- Kopčanová M, Tait L, Donoghue T, et al. Resting-state EEG signatures of Alzheimer’s disease are driven by periodic but not aperiodic changes. Neurobiol Dis. 2024;190. doi:10.1016/j.nbd.2023.106380
- Rodrigues P, Teixeira JP. Artificial Neural Networks in the Discrimination of Alzheimer’s Disease. Berlin, Heidelberg: Springer; 2011. p. 272-281. doi: 10.1007/978-3-642-24352-3_29
- Fiscon G, Weitschek E, Cialini A, et al. Combining EEG signal processing with supervised methods for Alzheimer’s patients classification. BMC Med Inform Decis Mak. 2018;18(1):35. doi: 10.1186/s12911-018-0613-y
- Kulkarni NN, Parhad SV, Shaikh YP. Use of Non-Linear and Complexity Features for EEG Based Dementia & Alzheimer Disease Diagnosis. United States: IEE; 2017. p. 1-3. doi: 10.1109/ICCUBEA.2017.8463870
- Peck R. Preliminary Edition of Statistics: Learning from Data (Book Only). United States: Cengage Learning; 2013.
- Richman JS, Moorman JR. Physiological time-series analysis using approximate entropy and sample entropy. Am J Physiol Heart Circ Physiol. 2000;278(6):H2039-H2049. doi: 10.1152/ajpheart.2000.278.6.H2039
- Bandt C, Pompe B. Permutation entropy: A natural complexity measure for time series. Phys Rev Lett. 2002;88(17):174102. doi: 10.1103/PhysRevLett.88.174102
- Wang Z, Alzheimer’s Disease Neuroimaging I. Brain entropy mapping in healthy aging and Alzheimer’s disease. Front Aging Neurosci. 2020;12:596122. doi: 10.3389/fnagi.2020.596122
- Jelinek HF, Donnan L, Khandoker AH. Singular value decomposition entropy as a measure of ankle dynamics efficacy in a Y-balance test following supportive lower limb taping. Annu Int Conf IEEE Eng Med Biol Soc. 2019;2019:2439-2442. doi: 10.1109/EMBC.2019.8856655
- Pincus SM. Approximate entropy as a measure of system complexity. Proc Natl Acad Sci. 1991;88(6):2297-2301. doi: 10.1073/pnas.88.6.2297
- Burdess N. Starting Statistics: A Short, Clear Guide. United States: SAGE Publications; 2010.
- Wang R, Yang Z, Wang J, Shi L. Power Spectral Density and Lempel-Ziv Complexity Analysis of EEG in Alzheimer’s Disease. In: Conference: 2017 36th Chinese Control Conference (CCC); 2017. p. 5549-5554. doi: 10.23919/ChiCC.2017.8028238
- García S, Luengo J, Herrera F. Data Preprocessing in Data Mining. Berlin: Springer International Publishing; 2014.
- Pedregosa F, Varoquaux G, Gramfort A, et al. Scikit-learn: Machine Learning in Python. J Mach Learn Res. 2011;12:2825-2830.
- Vakili M, Ghamsari M, Rezaei M. Performance Analysis and Comparison of Machine and Deep Learning Algorithms for IoT Data Classification. 2020. doi: 10.48550/arXiv.2001.09636
- Akrofi K, Pal R, Baker MC, Nutter BS, Schiffer RW. Classification of Alzheimer’s Disease and Mild Cognitive Impairment by Pattern Recognition of EEG Power and Coherence. In: Conference: Proceedings of the IEEE International Conference on Acoustics, Speech, and Signal Processing, ICASSP; 2010. p. 606-609. doi: 10.1109/ICASSP.2010.5495193
- Buscema M, Rossini P, Babiloni C, Grossi E. The IFAST model, a novel parallel nonlinear EEG analysis technique, distinguishes mild cognitive impairment and Alzheimer’s disease patients with high degree of accuracy. Artif Intell Med. 2007;40(2):127-141. doi: 10.1016/j.artmed.2007.02.006
- Huang C, Wahlund LO, Dierks T, Julin P, Winblad B, Jelic V. Discrimination of Alzheimer’s disease and mild cognitive impairment by equivalent EEG sources: A cross-sectional and longitudinal study. Clin Neurophysiol. 2000;111(11):1961-1967. doi: 10.1016/S1388-2457(00)00454-5
- Aghajani H, Zahedi E, Jalili M, Keikhosravi A, Vahdat BV. Diagnosis of early Alzheimer’s disease based on EEG source localization and a standardized realistic head model. IEEE J Biomed Health Inform. 2013;17(6):1039-45. doi: 10.1109/JBHI.2013.2253326
- Cunha RM, Silva G, Alves M, et al. EEG wavelet packet power spectrum tool for checking Alzheimer’s disease progression. Int J Biomed Eng Technol. 2022;40(3):289-302. doi: 10.1504/ijbet.2022.126497
- Melissant C, Ypma A, Frietman EE, Stam CJ. A method for detection of Alzheimer’s disease using ICA-enhanced EEG measurements. Artif Intell Med. 2005;33(3):209-222. doi: 10.1016/j.artmed.2004.07.003
- Khatun S, Morshed BI, Bidelman GM. A single-channel EEG-based approach to detect mild cognitive impairment via speech-evoked brain responses. IEEE Trans Neural Syst Rehabil Eng. 2019;27(5):1063-1070. doi: 10.1109/TNSRE.2019.2911970
- Petrosian AA, Prokhorov DV, Lajara-Nanson W, Schiffer RB. Recurrent neural network-based approach for early recognition of Alzheimer’s disease in EEG. Clin Neurophysiol. 2001;112(8):1378-1387. doi: 10.1016/s1388-2457(01)00579-x
- Pirrone D, Weitschek E, Di Paolo P, De Salvo S, De Cola MC. EEG signal processing and supervised machine learning to early diagnose Alzheimer’s disease. Appl Sci. 2022;12(11):5413. doi: 10.3390/app12115413
- Poil SS, de Haan W, van der Flier WM, Mansvelder HD, Scheltens P, Linkenkaer-Hansen K. Integrative EEG biomarkers predict progression to Alzheimer’s disease at the MCI stage. Front Aging Neurosci. 2013;5:58. doi: 10.3389/fnagi.2013.00058
- Vialatte F, Cichocki A, Dreyfus G, Musha T, Shishkin SL, Gervais R. Early Detection of Alzheimer’s Disease by Blind Source Separation, Time Frequency Representation, and Bump Modeling of EEG Signals. Berlin, Heidelberg: Springer; 2005. p. 683-692. doi: 10.1007/11550822_106
- Kulkarni N. Use of complexity based features in diagnosis of mild Alzheimer disease using EEG signals. Int J Inform Technol. 2017;10(1):59-64. doi: 10.1007/s41870-017-0057-0
- Rodrigues PM, Bispo BC, Garrett C, Alves D, Teixeira JP, Freitas D. Lacsogram: A new EEG tool to diagnose Alzheimer’s disease. IEEE J Biomed Health Inform. 2021;25(9):3384-3395. doi: 10.1109/JBHI.2021.3069789
- Dauwels J, Srinivasan K, Ramasubba Reddy M, et al. Slowing and loss of complexity in Alzheimer’s EEG: Two sides of the same coin? Int J Alzheimers Dis. 2011;2011:539621. doi: 10.4061/2011/539621
- Araujo T, Teixeira JP, Rodrigues PM. Smart-Data-driven system for Alzheimer disease detection through electroencephalographic signals. Bioengineering (Basel). 2022;9(4):141. doi: 10.3390/bioengineering9040141
- Tran XT, Le L, Nguyen QT, Do T, Lin CT. EEG-SSM: Leveraging State-Space Model for Dementia Detection. 2024. doi: 10.48550/arXiv.2407.17801
- Dogan S, Baygin M, Tasci B, et al. Primate brain pattern-based automated Alzheimer’s disease detection model using EEG signals. Cogn Neurodyn. 2023;17(3):647-659. doi: 10.1007/s11571-022-09859-2
- Jia H, Huang Z, Caiafa CF, et al. Assessing the potential of data augmentation in EEG functional connectivity for early detection of Alzheimer’s disease. Cogn Computat. 2023;16(1):229-242. doi: 10.1007/s12559-023-10188-7
- Yuda E, Ando T, Kaneko I, Yoshida Y, Hirahara D. Comprehensive data augmentation approach using WGAN-GP and UMAP for enhancing Alzheimer’s disease diagnosis. Electronics. 2024;13(18):3671. doi: 10.3390/electronics13183671