A multivariate statistical analysis of the effects of styrene maleic acid encapsulated RL71 in a xenograft model of triple negative breast cancer
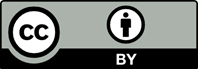
We have previously shown that the curcumin derivative 3,5-bis(3,4,5-trimethoxybenzylidene)-1-methylpiperidine-4-one (RL71), when encapsulated in styrene maleic acid micelles (SMA-RL71), significantly suppressed the growth of MDAMB-231 xenografts by 67%. Univariate statistical analysis showed that pEGFR/EGFR, pAkt/Akt, pmTOR/mTOR and p4EBP1/4EPBP1 were all significantly decreased in tumors from treated mice compared to SMA controls. In this study, multivariate statistical analyses (MVAs) were performed to identify the molecular networks that worked together to drive tumor suppression, with the aim to determine if this analysis could also be used to predict treatment outcome. Linear discriminant analysis correctly predicted, to 100% certainty, mice that received SMA-RL71 treatment. Additionally, results from multiple linear regression showed that the expression of Ki67, PKC-α, PP2AA-α, PP2AA-β and CaD1 networked together to drive tumor growth suppression. Overall, the MVAs provided evidence for a molecular network of signaling proteins that drives tumor suppression in response to SMA-RL71 treatment, which should be explored further in animal studies of cancer.
1. Somers-Edgar TJ, Taurin S, Larsen L, Chandramouli A, Nelson MA, and Rosengren RJ. (2011) Mechanisms for the activity of heterocyclic cyclohexanone curcumin derivatives in estrogen receptor negative human breast cancer cell lines. Invest New Drugs 29: 87-97.
2. Yadav B, Taurin S, Larsen L, and Rosengren RJ. (2012) RL66 a second-generation curcumin analog has potent in vivo and in vitro anticancer activity in ER‑negative breast cancer models. Int J Oncol 41: 1723-1732.
3. Yadav B, Taurin S, Larsen L, and Rosengren RJ. (2012) RL71, a second-generation curcumin analog, induces apoptosis and downregulates Akt in ER-negative breast cancer cells. Int J Oncol 41: 1119-1127.
4. Yadav B, Taurin S, Rosengren RJ, et al. (2010) Synthesis and cytotoxic potential of heterocyclic cyclohexanone analogues of curcumin. Bioorg Med Chem 18: 6701-6707.
5. Bisht S, Schlesinger M, Rupp A, Schubert R, Nolting J, Wenzel J, et al. (2016) A liposomal formulation of the synthetic curcumin analog EF24 (Lipo-EF24) inhibits pancreatic cancer progression: towards future combination therapies. J Nanobiotechnol 14: 57.
6. Nalli M, Ortar G, Moriello AS, Di Marzo V, and De Petrocellis L. (2017) Effects of curcumin and curcumin analogues on TRP channels. Fitoterapia 122: 126-131.
7. Padhye S, Banerjee S, Chavan D, Pandye S, Swamy KV, Ali S, et al. (2009) Fluorocurcumins as cyclooxygenase-2 inhibitor: molecular docking, pharmacokinetics and tissue distribution in mice. Pharm Res 26: 2438-2445.
8. Qiu X, Du Y, Lou B, Zuo Y, Shao W, Huo Y, et al. (2010) Synthesis and identification of new 4-arylidene curcumin analogues as potential anticancer agents targeting nuclear factor-κB signaling pathway. J Med Chem 53: 8260-8273.
9. Vyas A, Dandawate P, Padhye S, Ahmad A, & Sarkar F. (2013) Perspectives on new synthetic curcumin analogs and their potential anticancer properties. Cur Pharm Des 19: 2047-2069.
10. Greish K. (2010) Enhanced permeability and retention (EPR) effect for anticancer nanomedicine drug targeting. In: Cancer Nanotechnology. Springer, pp 25-37.
11. Taurin S, Nehoff H, Diong J, Larsen L, Rosengren RJ, and Greish K. (2013) Curcumin-derivative nanomicelles for the treatment of triple negative breast cancer. J Drug Tar 21: 675-683.
12. Angelova N, and Yordanov G. (2014) Nanopharmaceutical formulations based on poly (styrene-co-maleic acid). Bulg J Chem 3: 33-43.
13. Martey ONK, Nimick M, Taurin S, Sundararajan V, Greish K and Rosengren RJ. (2017) Styrene maleic acid-encapsulated RL71 micelles suppress tumour growth in a murine xenograft model of triple negative breast cancer. In J Nanomed 12: 7225-7237.
14. Manly BFJ. (2005) Multivariate statistical analyses. A Primer. 3rd Edition. London: Chapman and Hall/CRC.
15. Kitbumrungrat K. (2012) Comparison of logistic regression and discriminant analysis in classification groups for breast cancer. Int. J Comp Sci Net Security 12: 111-115.
16. Somers‐Edgar TJ, Scandlyn MJ, Stuart EC, Le Nedelec MJ, Valentine SP, and Rosengren RJ. (2008) The combination of epigallocatechin gallate and curcumin suppresses ERα‐breast cancer cell growth in vitro and in vivo. Int J Cancer 122: 1966-1971.
17. Brook RJ, Arnold GC. (1985) Applied regression analysis and experimental design. Boca Raton: Chapman and Hall/CRC.
18. Ryan TP. (2009) Modern regression methods. New Jersey: Wiley.
19. Stevens JP. (2009) Applied multivariate statistics for the social sciences. 5th Edition., Hillsdale NJ: Lawrence Erlbaum.
20. Noes T, Mevik B-H. (2001) Understanding the collinearity problem in regression and discriminant analysis. J. Chemometrics 15: 413-426.
21. Babayak MA. (2004) What you see may not be what you get: A brief, non-technical introduction to over-fitting in regression-type models. Psychosomat Med 66: 411-421.
22. Vittinghoff E, Glidden DV, Shiboski SC, McCulloch CE.(2005) Regression methods in statistics: Linear, logistic, survival and repeated measures models. New York: Springer.
23. Field A. (2011) Discovering statistics using SPSS. Los Angeles: Sage.
24. Smith PF. (2018) On the application of multivariate statistical and data mining analyses to data in neuroscience. J Undergrad Neurosci Educ 16: R20-R32.
25. Zavorka S, Perrett JJ. (2014) Minimum sample size considerations for two-group linear and quadratic discriminant analysis with rare populations. Communications in Statistics – Simulation and Computation. 43: 1726-1739.
26. Lachenbruch PA. (1968) On expected probabilities of misclassification in discriminant analysis, necessary sample size and a relation with the multiple correlation coefficient. Biometrics. 24(4): 823-834.
27. Breiman L, Friedman J, Stone CJ, and Olshen R. (1984) Classification and regression trees. 1st Edition. Boca Raton: CRC Press.
28. Pang H, Lin A, Holford M, Enerson BE, Lu B, Lawton MP, Floyd E, and Zhao H. (2006) Pathway analysis using random forests classification and regression. Bioinform 22: 2028-2036.
29. Hastie T, Tibshirani R, and Friedman J. (2009) Elements of statistical learning: Data mining, inference and prediction. 2nd Edition. Heidelberg: Springer Verlag.
30. Williams GJ. (2011) Data mining with Rattle and R. New York: Springer.
31. Gunduz N, Fokoue E. (2015) Robust classification of high dimension low sample size data. arXiv: 1501.00592v1.
32. Cueto-López N, García-Ordás MT, Dávila-Batista V, Moreno V, Aragonés N, Alaiz-Rodríguez R. (2019) A comparative study on feature selection for a risk prediction model for colorectal cancer. Comput Methods Programs Biomed. 177:219-229.
33. De Azambuja E, Cardoso F, de Castro Jr G, Colozza M, Mano MS, Durbecq V, et al. (2007) Ki-67 as prognostic marker in early breast cancer: a meta-analysis of published studies involving 12 155 patients. Br J Cancer 96: 1504.
34. Soliman NA, and Yussif SM. (2016) Ki-67 as a prognostic marker according to breast cancer molecular subtype. Cancer Bio Med 13: 496-504.
35. Xie Y, Chen L, Ma X, Li H, Gu L, Gao Y, et al. (2017) Prognostic and clinicopathological role of high Ki-67 expression in patients with renal cell carcinoma: a systematic review and meta-analysis. Scientific Rep 7: 44281.
36. Yerushalmi R, Woods R, Ravdin PM, Hayes MM, & Gelmon KA. (2010) Ki67 in breast cancer: prognostic and predictive potential. Lancet Oncol 11: 174-183.
37. International Breast Cancer Study Group. (2002) Endocrine responsiveness and tailoring adjuvant therapy for postmenopausal lymph node-negative breast cancer: a randomized trial. JNCI 94: 1054-1065.
38. Viale G, Regan MM, Mastropasqua MG, Maffini, F, Maiorano E, Colleoni M. et al. (2008) Predictive value of tumor Ki-67 expression in two randomized trials of adjuvant chemoendocrine therapy for node-negative breast cancer. JNCI 100: 207-212.
39. Hou Q, Tan HT, Lim KH, Lim TK, Khoo A, Tan IB, et al. (2013) Identification and functional validation of caldesmon as a potential gastric cancer metastasis-associated protein. J Proteo Res 12: 980-990.
40. Yoshio T, Morita T, Kimura Y, Tsujii M, Hayashi N, and Sobue K. (2007) Caldesmon suppresses cancer cell invasion by regulating podosome/invadopodium formation. FEBS Let 581: 3777-3782.
41. Shang S, Hua F, and Hu Z-W. (2017) The regulation of β-catenin activity and function in cancer: therapeutic opportunities. Oncotar 8: 33972.
42. Badr G, Gul HI, Yamali C, Mohamed AA, Badr BM, Gul M, et al. (2018) Curcumin analogue 1, 5-bis (4-hydroxy-3-((4-methylpiperazin-1-yl) methyl) phenyl) penta-1, 4-dien-3-one mediates growth arrest and apoptosis by targeting the PI3K/AKT/mTOR and PKC-theta signaling pathways in human breast carcinoma cells. Bioorgan Chem 78: 46-57.
43. Han X, Xu B, Beevers CS, Odaka Y, Chen L, Liu L, et al. (2012) Curcumin inhibits protein phosphatases 2A and 5, leading to activation of mitogen-activated protein kinases and death in tumor cells. Carcinogenesis 33: 868-875.
44. Yu N, Kakunda M, Pham V, Lill JR, Du P, Wongchenko M, et al. (2015) Hsp105 recruits protein phosphatase 2a to dephosphorylate β-catenin. Mol Cell Bio 35:1390-1400.
45. Shieh JM, Chen YC, Lin YC, Lin JN, Chen WC, Chen YY, et al. (2013) Demethoxycurcumin inhibits energy metabolic and oncogenic signaling pathways through AMPK activation in triple-negative breast cancer cells. J Agri Food Chem 61: 6366-6375.
46. Deininger Sr-O, Ebert MP, Futterer A, Gerhard M, and Röcken C. (2008) MALDI imaging combined with hierarchical clustering as a new tool for the interpretation of complex human cancers. J Proteo Res 7: 5230-5236.