Artificial intelligence for diagnosing bladder pathophysiology: An updated review and future prospects
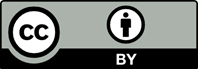
Background: Bladder pathophysiology encompasses a wide array of disorders, including bladder cancer, interstitial cystitis, overactive and underactive bladder, and bladder outlet obstruction. It also involves conditions such as neurogenic bladder, bladder infections, trauma, and congenital anomalies. Each of these conditions presents unique challenges for diagnosis and treatment. Recent advancements in artificial intelligence (AI) have shown significant potential in revolutionizing diagnostic methodologies within this domain. Objective: This review provides an updated and comprehensive examination of the integration of AI into the diagnosis of bladder pathophysiology. It highlights key AI techniques, including machine learning and deep learning, and their applications in identifying and classifying bladder conditions. The review also assesses current AI-driven diagnostic tools, their accuracy, and clinical utility. Furthermore, it explores the challenges and limitations confronted in the implementation of AI technologies, such as data quality, interpretability, and integration into clinical workflows, among others. Finally, the paper discusses future directions and advancements, proposing pathways for enhancing AI applications in bladder pathophysiology diagnosis. This review aims to provide a valuable resource for clinicians, researchers, and technologists, fostering an in-depth understanding of AI’s roles and potential in transforming bladder disease diagnosis. Conclusion: While AI demonstrates considerable promise in enhancing the diagnosis of bladder pathophysiology, ongoing progresses in data quality, algorithm interpretability, and clinical integration are essential for maximizing its potential. The future of AI in bladder disease diagnosis holds great promise, with continued innovation and collaboration opening the possibility of more accurate, efficient, and personalized care for patients.
- O’Kane M, Rantell A. Intrapartum and postpartum bladder management: The poor relation of modern obstetrics. Obstet Gynaecol Reprod Med. 2023;33(7):190-196. doi: 10.1016/j.ogrm.2023.04.002
- Humphrey PA. Urinary bladder pathology 2004: An update. Ann Diagn Pathol. 2004;8(6):380-389. doi: 10.1053/j.anndiagpath.2004.08.012
- Mahapatra C, Thakkar R. In silico electrophysiological investigation of transient receptor potential melastatin-4 ion channel biophysics to study detrusor overactivity. Int J Mol Sci. 2024;25(13):6875. doi: 10.3390/ijms25136875
- Gilyazova I, Enikeeva K, Rafikova G, et al. Epigenetic and immunological features of bladder cancer. Int J Mol Sci. 2023;24(12):9854. doi: 10.3390/ijms24129854
- Mahapatra C, Brain K, Manchanda R. Biophysically realistic models of detrusor ion channels: Role in shaping spike and excitability. In: Urinary Bladder Physiology: Computational Insights. Delhi: Narosa Publishing House; 2024.
- Wagg A, Ackerman AL, Lai HH, Newman DK. Review of the impact and burden of urinary urgency on adults with overactive bladder. Int J Clin Pract. 2024;2024(1):5112405. doi: 10.1155/2024/5112405
- Hutchinson A, Nesbitt A, Joshi A, Clubb A, Perera M. Overactive bladder syndrome: Management and treatment options. Aust J Gen Pract. 2020;49(9):593-598. doi: 10.31128/ajgp-11-19-5142
- Tran L, Xiao JF, Agarwal N, Duex JE, Theodorescu D. Advances in bladder cancer biology and therapy. Nat Rev Cancer. 2021;21(2):104-121. doi: 10.1038/s41568-020-00313-1
- Pyrgidis N, Schulz GB, Ebner B, et al. Radical cystectomy with ileal orthotopic neobladder after 70 years leads to worse health-related quality of life. J Clin Med. 2024;13(20):6102. doi: 10.3390/jcm13206102
- Kanno T, Inoue T, Kawakita M, et al. Perioperative and oncological outcomes of laparoscopic radical cystectomy with intracorporeal versus extracorporeal ileal conduit: A matched-pair comparison in a multicenter cohort in Japan. Int J Urol. 2020;27(6):559-565. doi: 10.1111/iju.14245
- Bahlburg H, Hellmann T, Tully K, et al. Psychosocial distress and quality of life in patients after radical cystectomy-one year follow-up in 842 German patients. J Cancer Surviv. 2024;18(5):1600-1607. doi: 10.1007/s11764-023-01400-6
- Sarker IH. AI-based modeling: Techniques, applications and research issues towards automation, intelligent and smart systems. SN Comput Sci. 2022;3(2):158. doi: 10.1007/s42979-022-01043-x
- Joshi A, Mishra S, Jain D, Ludhiani S, Rajput A. Artificial intelligence: An emerging technique in pharmaceutical and healthcare systems. In: Bioinformatics Tools and Big Data Analytics for Patient Care. United States: Chapman and Hall, CRC; 2022. p. 71-90. doi: 10.1201/9781003226949-5
- Aldahiri A, Alrashed B, Hussain W. Trends in using IoT with machine learning in health prediction system. Forecasting. 2021;3(1):181-206. doi: 10.3390/forecast3010012
- Ahmad Z, Rahim S, Zubair M, Abdul-Ghafar J. Artificial intelligence (AI) in medicine, current applications and future role with special emphasis on its potential and promise in pathology: Present and future impact, obstacles including costs and acceptance among pathologists, practical and philosophical considerations. Diagn Pathol. 2021;16(1):24. doi: 10.1186/s13000-021-01085-4
- McKinney SM, Sieniek M, Godbole V, et al. International evaluation of an AI system for breast cancer screening. Nature. 2020;577(7788):89-94. doi: 10.1038/s41586-019-1799-6
- Schork NJ. Artificial intelligence and personalized medicine. In: Precision Medicine in Cancer Therapy. United States: John Wiley;2019. p. 265-283. doi: 10.1007/978-3-030-16391-4-11
- Khizir L, Bhandari V, Kaloth S, et al. From diagnosis to precision surgery: The transformative role of artificial intelligence in urologic imaging. J Endourol. 2024;38(8):824-835. doi: 10.1089/end.2023.0695
- Shkolyar E, Zhou SR, Carlson CJ, et al. Optimizing cystoscopy and TURBT: Enhanced imaging and artificial intelligence. Nat Rev Urol. 2024; 22:46-54. doi: 10.1038/s41585-024-00904-9
- Schulz A, Loloi J, Martina LP, Sankin A. The development of non-invasive diagnostic tools in bladder cancer. Onco Targets Ther. 2022;15:497-507. doi: 10.2147/ott.s283891
- Atkinson LZ, Cipriani A. How to carry out a literature search for a systematic review: A practical guide. BJPsych Adv. 2018;24(2):74-82. doi: 10.1192/bja.2017.3
- Rubilotta E. Bladder outlet obstruction and overactive bladder in males. In: Non-Neurogenic Bladder Dysfunctions. Germany: Springer Nature; 2021. p. 67-85. doi: 10.1007/978-3-030-57393-5-6
- Corcos J, Przydacz M. Pathologies responsible for the development of the neurogenic bladder. In: Consultation in Neurourology: A Practical Evidence-Based Guide. Germany: Springer; 2018. p. 17-36. doi: 10.1007/978-3-319-63910-9-3
- Chuang YC, Chermansky C, Kashyap M, Tyagi P. Investigational drugs for bladder pain syndrome (BPS)/interstitial cystitis (IC). Expert Opin Investig Drugs. 2016;25(5):521-529. doi: 10.1517/13543784.2016.1162290
- Dyrskjøt L, Hansel DE, Efstathiou JA, et al. Bladder cancer. Nat Rev Dis Primers. 2023;9(1):58. doi: 10.1038/s41572-023-00468-9
- Babjuk M, Burger M, Capoun O, et al. European association of urology guidelines on non-muscle-invasive bladder cancer (Ta, T1, and carcinoma in situ). Eur Urol. 2022;81(1):75-94. doi: 10.1016/j.eururo.2021.08.010
- Rouprêt M, Seisen T, Birtle AJ, et al. European association of urology guidelines on upper urinary tract urothelial carcinoma: 2023 update. Eur Urol. 2023;84(1):49-64. doi: 10.1016/j.eururo.2023.03.013
- Mahapatra C, Brain KL, Manchanda R. A biophysically constrained computational model of the action potential of mouse urinary bladder smooth muscle. PLoS One. 2018;13(7):e0199912. doi: 10.1371/journal.pone.0200712
- Kalejaiye O, Vij M, Drake MJ. Classification of stress urinary incontinence. World J Urol. 2015;33:1215-1220. doi: 10.1007/s00345-015-1617-1
- McCarthy TC. Cystoscopy. In: Veterinary Endoscopy for the Small Animal Practitioner. United States: John Wiley; 2021. p. 217-361. doi: 10.1002/9781119155904.ch6
- Moran PA, Cole J, Greenwell T. Cystoscopy and vesicoscopy. In: Textbook of Female Urology and Urogynecology. United States: CRC Press; 2023. p. 373-386. doi: 10.1201/9781003144236-41
- Wen JG. Cystometry, pressure flow study and urethral pressure measurement. In: Clinical Urodynamics in Childhood and Adolescence. Germany: Springer; 2018. p. 73-93. doi: 10.1007/978-3-319-42193-3-8
- Artibani W, Andersen JT, Gajewski JB, et al. Imaging and other investigations. In: Incontinence. 2nd ed. New York: Health Publication Ltd.; 2002. p. 425-477.
- Chudobiński C, Świderski B, Antoniuk I, Kurek J. Enhancements in radiological detection of metastatic lymph nodes utilizing AI-assisted ultrasound imaging data and the lymph node reporting and data system scale. Cancers(Basel). 2024;16(8):1564. doi: 10.3390/cancers16081564
- Eismann L, Rodler S, Tamalunas A, et al. Computed-tomography based scoring system predicts outcome for clinical lymph node-positive patients undergoing radical cystectomy. Int Braz J Urol. 2022;48(1):89-98. doi: 10.1590/S1677-5538.IBJU.2021.0329
- Salmanoglu E, Halpern E, Trabulsi EJ, Kim S, Thakur ML. A glance at imaging bladder cancer. Clin Transl Imaging. 2018;6(4):257-269. doi: 10.1007/s40336-018-0284-9
- Pavlica P, Gaudiano C, Barozzi L. Sonography of the bladder. World J Urol. 2004;22(5):328-334. doi: 10.1007/s00345-004-0415-y
- Bashkami A, Nasayreh A, Makhadmeh SN, et al. A review of artificial intelligence methods in bladder cancer: Segmentation, classification, and detection. Artif Intell Rev. 2024;57(12):339. doi: 10.1007/s10462-020-09844-1
- Castro-Olvera G, Baria E, Stoliarov D, et al. A review of label-free photonics-based techniques for cancer detection in the digestive and urinary systems. J Phys Photonics. 2024;7(1):25. doi: 10.1088/2515-7647/ad8613
- Zhuang YT, Wu F, Chen C, Pan YH. Challenges and opportunities: From big data to knowledge in AI 2.0. Front Inf Technol Electron Eng. 2017;18:3-14. doi: 10.1631/FITEE.1601883
- Ahmed I, Jeon G, Piccialli F. From artificial intelligence to explainable artificial intelligence in industry 4.0: A survey on what, how, and where. IEEE Trans Ind Inform. 2022;18(8):5031-5042. doi: 10.1109/tii.2022.3146552
- Alloghani M, Al-Jumeily D, Mustafina J, Hussain A, Aljaaf AJ. A systematic review on supervised and unsupervised machine learning algorithms for data science. In: Supervised and Unsupervised Learning for Data Science. Germany: Springer Nature; 2020. p. 3-21. doi: 10.1007/978-3-030-22475-2-1
- Sarker IH. Deep cybersecurity: A comprehensive overview from neural network and deep learning perspective. SN Comput Sci. 2021;2(3):154. doi: 10.1007/s42979-021-00535-6
- Liu W, Wang Z, Liu X, Zeng N, Liu Y, Alsaadi FE. A survey of deep neural network architectures and their applications. Neurocomputing. 2017;234:11-26. doi: 10.1016/j.neucom.2016.12.038
- Woerl AC, Eckstein M, Geiger J, et al. Deep learning predicts molecular subtype of muscle-invasive bladder cancer from conventional histopathological slides. Eur Urol. 2020;78(2):256-264. doi: 10.1016/j.eururo.2020.04.023
- Fernandez-Gomez J, Madero R, Solsona E, et al. Predicting nonmuscle invasive bladder cancer recurrence and progression in patients treated with bacillus calmette-guerin: The CUETO scoring model. J Urol. 2009;182(5):2195-2203. doi: 10.1016/j.juro.2009.07.016
- Eun SJ, Kim J, Kim KH. Applications of artificial intelligence in urological setting: A hopeful path to improved care. J Exerc Rehabil. 2021;17(5):308-312. doi: 10.12965/jer.2142596.298
- Borhani S, Borhani R, Kajdacsy-Balla A. Artificial intelligence: A promising frontier in bladder cancer diagnosis and outcome prediction. Crit Rev Oncol Hematol. 2022;171:103601. doi: 10.1016/j.critrevonc.2022.103601
- Khoraminia F, Fuster S, Kanwal N, et al. Artificial intelligence in digital pathology for bladder cancer: Hype or hope? A systematic review. Cancers. 2023;15(18):4518. doi: 10.3390/cancers15184518
- Ferro M, Falagario UG, Barone B, et al. Artificial intelligence in the advanced diagnosis of bladder cancer-comprehensive literature review and future advancement. Diagnostics(Basel). 2023;13(13):2308. doi: 10.3390/diagnostics13132308
- Jelovsek JE, Chagin K, Brubaker L, et al. A model for predicting the risk of de novo stress urinary incontinence in women undergoing pelvic organ prolapse surgery. Obstet Gynecol. 2014;123(2 Pt 1):279-287. doi: 10.1097/aog.0000000000000094
- Sinha S, Sinha U, Malis V, Bhargava V, Sakamoto K, Rajasekaran E. Exploration of male urethral sphincter complex using diffusion tensor imaging (DTI)-based fiber-tracking. J Magn Reson Imaging. 2018;48(4):1002-1011. doi: 10.1002/jmri.26017
- Kim ES, Eun SJ, Kim KH. Artificial intelligence-based patient monitoring system for medical support. Int Neurourol J. 2023;27(4):280-286. doi: 10.5213/inj.2346338.169
- Zhou T, Zhu C, Zhang W, et al. Identification and validation of immune and diagnostic biomarkers for interstitial cystitis/ painful bladder syndrome by integrating bioinformatics and machine-learning. Front Immunol. 2025;16:1511529. doi: 10.3389/fimmu.2025.1511529
- Klen R, Salminen AP, Mahmoudian M, et al. Prediction of complication related death after radical cystectomy for bladder cancer with machine learning methodology. Scand J Urol. 2019;53(5):325-331. doi: 10.1080/21681805.2019.1665579
- Tan J, Qin F, Yuan J. Current applications of artificial intelligence combined with urine detection in disease diagnosis and treatment. Transl Androl Urol. 2021;10(4):1769-1779. doi: 10.21037/tau-20-1405
- Mei H, Wang Z, Zheng Q, et al. Deep learning and numerical analysis for bladder outflow obstruction and detrusor underactivity diagnosis in men: A novel urodynamic evaluation scheme. Neurourol Urodyn. 2025:44(2):512-519. doi: 10.1002/nau.25665
- Lei T, Zhao X, Jin S, Meng Q, Zhou H, Zhang M. Discovery of potential bladder cancer biomarkers by comparative urine proteomics and analysis. Clin Genitourin Cancer. 2013;11(1):56-62. doi: 10.1016/j.clgc.2012.06.003
- Wan X, Wang D, Zhang X, et al. Unleashing the power of urine-based biomarkers in diagnosis, prognosis and monitoring of bladder cancer. Int J Oncol. 2025;66(3):18. doi: 10.3892/ijo.2025.5724
- Zheng Y, Gan W, Chen Z, Qi Z, Liang Q, Yu PS. Large language models for medicine: A survey. Int J Mach Learn Cybern. 2024;16(2):1-26. doi: 10.1007/s13042-024-02318-w
- Solano C, Tarazona N, Angarita GP, et al. ChatGPT in urology: Bridging knowledge and practice for tomorrow’s healthcare, a comprehensive review. J Endourol. 2024;38(8):763-777. doi: 10.1089/end.2023.0700
- Coskun B, Ocakoglu G, Yetemen M, Kaygisiz O. Can ChatGPT, an artificial intelligence language model, provide accurate and high-quality patient information on prostate cancer? Urology. 2023;180:35-58. doi: 10.1016/j.urology.2023.05.040
- Hu M, Qian J, Pan S, Li Y, Qiu RLJ, Yang X. Advancing medical imaging with language models: Featuring a spotlight on ChatGPT. Phys Med Biol. 2024;69(10):10TR01. doi: 10.1088/1361-6560/ad387d
- Wischmeyer T. Artificial intelligence and transparency: Opening the black box. In: Regulating Artificial Intelligence. Germany: Springer Nature; 2020. p. 75-101. doi: 10.1007/978-3-030-32361-5-4
- Isibor E. Regulation of healthcare data security: Legal obligations in a digital age. SSRN. 2024;18:132. doi: 10.2139/ssrn.4957244
- Oseni A, Moustafa N, Janicke H, et al. Security and privacy for artificial intelligence: Opportunities and enges. Arxiv. 2021. Preprint. doi: 10.48550/arXiv.2102.04661